Rephrase and rearrange the whole content into a news article. I want you to respond only in language English. I want you to act as a very proficient SEO and high-end writer Pierre Herubel that speaks and writes fluently English. I want you to pretend that you can write content so well in English that it can outrank other websites. Make sure there is zero plagiarism.:
A new artificial intelligence system that predicts the onset of psychosis before it strikes holds the potential to revolutionize early detection in mental health care.
Paranoia, disorganized thinking, hallucinations, or sudden changes are a few among the many ways a psychotic episode can take shape. It has been estimated that over 5% of people will experience a psychosis event at some point in their lifetime. Advances in neuroimaging techniques, like magnetic resonance imaging or MRI, have enabled researchers to examine with greater detail the structural and chemical underpinnings of psychiatric diseases, which were previously less understood.
Shinsuke Koike, the corresponding author of a Molecular Psychiatry study and Associate professor at the Graduate School of Arts and Sciences at the University of Tokyo, said inan interview with Tech Times that the performance of the new system “established machine learning’s ability to differentiate between MRI brain scans of people at high risk of psychosis and healthy controls.”
The Link Between Psychosis and Brain Structure
Research using structural MRI scans has found a connection between a higher risk for psychosis and changes in brain structure. People moving from a high-risk state to psychosis showed a gradual decrease in gray matter volume in certain brain areas. Similarly, thinner cortex has been linked to schizophrenia or the start of psychosis symptoms.
Building on the understanding that changes in brain structure can forecast real-life outcomes, the researchers in this study taught a machine-learning (ML) classifier to recognize patterns in participants’ brain anatomy. Using a dataset of MRI brain scans, including 120 clinically high-risk individuals who developed psychosis and 799 healthy individuals, the ML algorithm was trained. Remarkably, the classifier accurately distinguished between healthy individuals and those at risk of psychosis, achieving an impressive accuracy rate of 85%.
A dataset of 2000 MRI brain scans of young adult and adolescent participants -1165 at high risk and 1029 healthy individuals-was collected from 21 sites across 15 different countries to test its prediction ability. For a better analysis of brain changes across various stages of psychosis development, the participants of the high-risk group were divided into three categories: those who developed psychosis, those who did not develop psychosis, and those with uncertain follow-up status.
At this stage, the researchers encountered a unique challenge: harmonizing all brain scan data collected from multiple sources into a composite dataset to train the ML algorithm. Koike pointed out that MRI research of psychotic disorders is already complex due to significant variations in brain development across populations. Disparities in the making and building of MRI machines just added to the existing difficulties. “It’s like taking a picture with a digital camera, which could be an iPhone, iPad, or an Android. Even if you click a picture of the same thing, the take-home photograph is quite different. Similarly, in MRI, different machines, different protocols, and different parameters give rise to different brain scan images,” he added.
The data from 21 sites were harmonized by ENIGMA CHR Working Group- an international group that investigates structural brain alterations in those at clinically high risk for psychosis- using ComBat, a statistical tool.
Primed and ready with a consolidated dataset, the classifier compared brain scans, identified the healthy controls, and predicted the onset of psychosis before it occurred with a striking 73% accuracy!
Adolescent development ushers in a string of bodily changes, including a brain-wide decline in gray matter volume, cortical surface area, and cortical thickness by about 10%. Thus, the time frame from adolescence to early adulthood is a high-risk period for the development of psychosis. Often the most common age for a first psychotic episode. This brief transition period, therefore, presents a crucial window for intervention and prevention of psychosis.
Machine learning approaches have shown proficiency in identifying individuals with schizophrenia based on subtle differences in brain structures appearing on MRI brain scans but haven’t been able to identify cases before their occurrence.
Brain development patterns of adolescents with a high risk of psychosis differ from those of healthy individuals, with the former group exhibiting altered non-linear age associations with cortical thickness. The new ML classifier tool, built upon these insights, analyzes nuances in MRI brain scans and interprets said knowledge to predict the risk of psychosis before its onset. It provided researchers with a handy tool for the development of early intervention techniques that can identify adolescents who need help dealing with psychosis and its symptoms.
Future Prospects For Mental Health Diagnoses
The increase in public discourse around mental health issues and disorders over the last couple of decades has led to significant progress in the field of psychiatry. However, there is a lack of clinical methods like blood tests for identifying mental ailments, nor are there any clear guidelines for the use of neuroimaging for diagnosis.
With no biological markers to rely on, the onus of identifying whether a person will go on to develop symptoms of psychosis falls entirely on the clinicians or psychiatrists. The clinician has to meet the patient, talk about their difficulties, and then, based on subclinical signs, such as changes in behavior, emotion, and thinking ability, decide whether an individual is at high risk or healthy, said Koike.
While the classifier’s scope is currently confined to the training and test datasets, the findings represent a significant milestone. They mark one of the earliest instances where machine learning accurately pinpointed brain differences between healthy individuals and those at high risk of psychosis but have not yet experienced it. Koike and the team are now focused on refining classifiers capable of handling new datasets, aiming to integrate them into everyday clinical practice.
ⓒ 2024 TECHTIMES.com All rights reserved. Do not reproduce without permission.
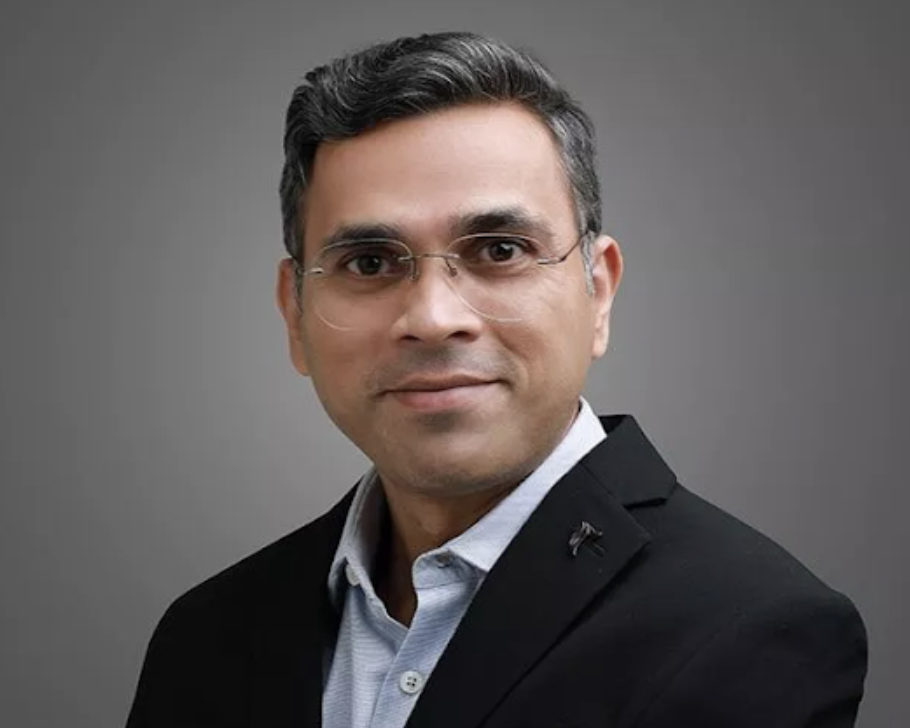
I have over 10 years of experience in the cryptocurrency industry and I have been on the list of the top authors on LinkedIn for the past 5 years. I have a wealth of knowledge to share with my readers, and my goal is to help them navigate the ever-changing world of cryptocurrencies.